class: center middle main-title section-title-1 # Causal Assumptions .class-info[ **Session 4** .light[STA 379/679: Causal Inference <br> Lucy D'Agostino McGowan ] ] --- class: title title-1 # Dichotomous exposure & outcome .box-inv-1.medium[X (1: exposed, 0: unexposed)] -- .box-inv-1.medium[Y (1: died, 0: survived)] --- class: title title-1 center # Potential Outcome <br> <br> .box-1[ What *will* happen to you in the future given you have a particular exposure ] <br> -- .box-inv-1.medium[ **fixed** but **unknown** ] --- class: title title-1 center # Potential Outcomes .box-1.medium[ `\(\large Y(x)\)` ] <br> -- .box-inv-1.medium[ The outcome `\((Y)\)` under a specific exposure `\((X=x)\)` ] --- class: title title-1 center # Potential Outcomes .box-1.medium[ `\(\large Y(1)\)` ] <br> -- .box-inv-1.medium[ The outcome `\((Y)\)` with exposure `\((X=1)\)` ] --- class: title title-1 center # Potential Outcomes .box-1.medium[ `\(\large Y(0)\)` ] <br> -- .box-inv-1.medium[ The outcome `\((Y)\)` without exposure `\((X=0)\)` ] --- class: title title-1 center # Potential Outcomes .box-1.medium[ `\(\large Y(1) = 0\)` ] <br> -- .box-inv-1.medium[ The outcome `\((Y)\)` with exposure `\((X=1)\)` is **"survival"** ] --- class: title title-1 center # Potential Outcomes .box-1.medium[ `\(\large Y(0) = 1\)` ] <br> -- .box-inv-1.medium[ The outcome `\((Y)\)` without exposure `\((X=0)\)` is **"death"** ] --- class: title title-1 # Potential Outcomes .box-inv-1.medium[ Will eating ice cream make me happy? ] -- .box-1.medium[Four options:] (1) I am happy only with ice cream -- .box-inv-1[ `\(Y\)`(π¦) = π, `\(Y\)`(no π¦) = π ] --- class: title title-1 # Potential Outcomes .box-inv-1.medium[ Will eating ice cream make me happy? ] .box-1.medium[Four options:] (2) No effect of ice cream, I am happy either way -- .box-inv-1[ `\(Y\)`(π¦) = π, `\(Y\)`(no π¦) = π ] --- class: title title-1 # Potential Outcomes .box-inv-1.medium[ Will eating ice cream make me happy? ] .box-1.medium[Four options:] (3) No effect of ice cream, I am angry either way -- .box-inv-1[ `\(Y\)`(π¦) = π , `\(Y\)`(no π¦) = π ] --- class: title title-1 # Potential Outcomes .box-inv-1.medium[ Will eating ice cream make me happy? ] .box-1.medium[Four options:] (4) I am happy only without ice cream -- .box-inv-1[ `\(Y\)`(π¦) = π , `\(Y\)`(no π¦) = π ] --- class: title title-1 # Potential Outcomes .pull-left[ .box-1.medium[No Causal Effect] <br> .box-inv-1[ `\(Y\)`(π¦) = π , `\(Y\)`(no π¦) = π ] <br> .box-inv-1[ `\(Y\)`(π¦) = π, `\(Y\)`(no π¦) = π ] ] -- .pull-right[ .box-1.medium[Causal Effect] <br> .box-inv-1[ `\(Y\)`(π¦) = π, `\(Y\)`(no π¦) = π ] <br> .box-inv-1[ `\(Y\)`(π¦) = π , `\(Y\)`(no π¦) = π ] ] --- class: title title-1 # Potential Outcome <br> <br> .box-1[ What *will* happen to you in the future given you have a particular exposure ] <br> -- .box-inv-1.medium[ **fixed** but **unknown** ] --- class: title title-1 # What is a causal effect .box-inv-1.medium[The causal effect is the comparison of the potential outcomes for the same unit at the same moment in time post-exposure] -- .box-inv-1.medium[Whether the effect is **causal** depends on the potential outcomes but it **does not** depend on which outcome is actually observed] --- class: title title-1 center # Outcome for an individual .box-1.medium[ Individual *i* has a pair of potential outcomes ] <br> -- .box-inv-1.medium[ `\((Y_i(1), Y_i(0))\)` ] --- class: title title-1 center # Outcome for an individual .box-1.medium[ Individual *i* has a pair of potential outcomes ] <br> .box-inv-1.medium[ `\(Y_i\)`(π¦), `\(Y_i\)`(no π¦) ] --- class: title title-1 # Causal Effect .box-inv-1.medium[ `\(\Huge Y(1) - Y(0)\)` ] --- class: title title-1 # Causal Effect .box-inv-1.medium[ `\(\Huge Y_i(1) - Y_i(0)\)` ] --- class: title title-1 # Causal Estimand .box-inv-1.medium[ `$$\large \frac{1}{N}\sum_{i=1}^N(Y_i(1) - Y_i(0))$$` ] --- class: title title-1 # Potential Outcome <br> <br> .box-1[ What *will* happen to you in the future given you have a particular exposure ] <br> -- .box-inv-1.medium[ again...**fixed** but **unknown** ] --- class: title title-1 # What do we observe? .box-inv-1.medium[Recall: The causal effect is the comparison of the potential outcomes for the same unit at the same moment in time post-exposure] --- class: title title-1 # What do we observe? .box-inv-1.medium[Recall: The causal effect is the comparison of the potential outcomes for the same unit **at the same moment** in time post-exposure] -- .center[ 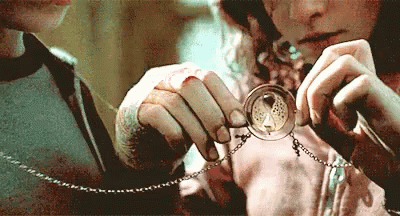 ] --- class: title title-1 # Observed outcome for an individual .pull-left[ .box-inv-1[ Individual `\(i\)` eats π¦ ] ] --- class: title title-1 # Observed outcome for an individual .pull-left[ .box-inv-1[ Individual `\(i\)` eats π¦ ] <br> .box-1.medium[ `\(Y_i^{obs}\)`(π¦) ] ] -- .pull-right[ .box-inv-1[ Individual `\(i\)` does **not** eat π¦ ] ] --- class: title title-1 # Observed outcome for an individual .pull-left[ .box-inv-1[ Individual `\(i\)` eats π¦ ] <br> .box-1.medium[ `\(Y_i^{obs}\)`(π¦) ] ] .pull-right[ .box-inv-1[ Individual `\(i\)` does **not** eat π¦ ] <br> .box-1.medium[ `\(Y_i^{obs}\)`(no π¦) ] ] --- class: title title-1 # Observed outcome for an individual .pull-left[ .box-inv-1[ Individual `\(i\)` eats π¦ ] <br> .box-1.medium[ `\(Y_i^{obs}\)`(π¦) ] ] .pull-right[ .box-2[ Individual `\(i\)` does **not** eat π¦ ] <br> .box-2.medium[ `\(Y_i^{obs}\)`(no π¦) ] ] --- class: title title-1 # Observed outcome for an individual .pull-left[ .box-2[ Individual `\(i\)` eats π¦ ] <br> .box-2.medium[ `\(Y_i^{obs}\)`(π¦) ] ] .pull-right[ .box-inv-1[ Individual `\(i\)` does **not** eat π¦ ] <br> .box-1.medium[ `\(Y_i^{obs}\)`(no π¦) ] ] --- class: title title-1 # Missing outcome for an individual .pull-left[ .box-inv-1[ Individual `\(i\)` eats π¦ ] ] --- class: title title-1 # Missing outcome for an individual .pull-left[ .box-inv-1[ Individual `\(i\)` eats π¦ ] <br> .box-1.medium[ `\(Y_i^{mis}\)`(no π¦) ] ] -- .pull-right[ .box-inv-1[ Individual `\(i\)` does **not** eat π¦ ] ] --- class: title title-1 # Missing outcome for an individual .pull-left[ .box-inv-1[ Individual `\(i\)` eats π¦ ] <br> .box-1.medium[ `\(Y_i^{mis}\)`(no π¦) ] ] .pull-right[ .box-inv-1[ Individual `\(i\)` does **not** eat π¦ ] <br> .box-1.medium[ `\(Y_i^{mis}\)`(π¦) ] ] --- class: title title-1 # Missing outcome for an individual .pull-left[ .box-inv-1[ Individual `\(i\)` eats π¦ ] <br> .box-1.medium[ `\(Y_i^{mis}\)`(no π¦) ] ] .pull-right[ .box-2[ Individual `\(i\)` does **not** eat π¦ ] <br> .box-2.medium[ `\(Y_i^{mis}\)`(π¦) ] ] --- class: title title-1 # Missing outcome for an individual .pull-left[ .box-2[ Individual `\(i\)` eats π¦ ] <br> .box-2.medium[ `\(Y_i^{mis}\)`(no π¦) ] ] .pull-right[ .box-inv-1[ Individual `\(i\)` does **not** eat π¦ ] <br> .box-1.medium[ `\(Y_i^{mis}\)`(π¦) ] ] --- class: title title-1 # Outcomes for an individual .box-inv-1[ `\(Y_i^{obs}= Y_i(X_i)=\begin{cases}Y_i(0)&\textrm{if }X_i=0\\Y_i(1)&\textrm{if }X_i = 1\end{cases}\)` ] -- <br> .box-1[ `\(Y_i^{mis}= Y_i(1-X_i)=\begin{cases}Y_i(1)&\textrm{if }X_i=0\\Y_i(0)&\textrm{if }X_i = 1\end{cases}\)` ] --- class: section-title-1 title-1 middle # Causal inference is fundamentally a missing data problem .footer[Rubin (1974) Journal of Educational Psychology doi:10.1037/h0037350] --- class: title title-1 # Does ice cream make us happy? .box-inv-1[Without a time turner, how can we try to answer our ice cream // happiness question?] -- .box-1.medium[Let's add some data!] --- class: title title-1 # Does ice cream make us happy? .pull-left[ .box-inv-1.medium[My possibilities] ] --- class: title title-1 # Does ice cream make us happy? .pull-left[ .box-inv-1.medium[My possibilities] <br> .box-1.small[ `\(Y_{me}\)`(π¦) = π, `\(Y_{me}\)`(no π¦) = π ] .box-1.small[ `\(Y_{me}\)`(π¦) = π, `\(Y_{me}\)`(no π¦) = π ] .box-1.small[ `\(Y_{me}\)`(π¦) = π , `\(Y_{me}\)`(no π¦) = π ] .box-1.small[ `\(Y_{me}\)`(π¦) = π , `\(Y_{me}\)`(no π¦) = π ] ] -- .pull-right[ .box-inv-1.medium[Your possibilities] <br> ] --- class: title title-1 # Does ice cream make us happy? .pull-left[ .box-inv-1.medium[My possibilities] <br> .box-1.small[ `\(Y_{me}\)`(π¦) = π, `\(Y_{me}\)`(no π¦) = π ] .box-1.small[ `\(Y_{me}\)`(π¦) = π, `\(Y_{me}\)`(no π¦) = π ] .box-1.small[ `\(Y_{me}\)`(π¦) = π , `\(Y_{me}\)`(no π¦) = π ] .box-1.small[ `\(Y_{me}\)`(π¦) = π , `\(Y_{me}\)`(no π¦) = π ] ] .pull-right[ .box-inv-1.medium[Your possibilities] <br> .box-1.small[ `\(Y_{you}\)`(π¦) = π, `\(Y_{you}\)`(no π¦) = π ] .box-1.small[ `\(Y_{you}\)`(π¦) = π, `\(Y_{you}\)`(no π¦) = π ] .box-1.small[ `\(Y_{you}\)`(π¦) = π , `\(Y_{you}\)`(no π¦) = π ] .box-1.small[ `\(Y_{you}\)`(π¦) = π , `\(Y_{you}\)`(no π¦) = π ] ] --- class: title-1 title # Does ice cream make us happy? .box-inv-1.medium[Exposure possibilities] -- .box-1.medium[We both eat ice cream] -- .box-1.medium[We both do not eat ice cream] -- .box-1.medium[I eat ice cream you do not] -- .box-1.medium[I do not eat ice cream, you do] --- class: title title-1 # Potential Outcomes .box-1[ `\(Y_{me}\)`(π¦, π¦), `\(Y_{me}\)`(no π¦, noπ¦), `\(Y_{me}\)`(π¦, no π¦), `\(Y_{me}\)`(no π¦, π¦) ] -- <br> .box-1[ `\(Y_{you}\)`(π¦, π¦), `\(Y_{you}\)`(no π¦, noπ¦), `\(Y_{you}\)`(π¦, no π¦), `\(Y_{you}\)`(no π¦, π¦) ] --- class: title title-1 ## Stable Unit Treatment Value Assumption .box-inv-1[ The potential outcomes for any unit do not vary with the exposures assigned to other units, and, for each unit, there are no different forms or versions of each exposure level, which lead to different potential outcomes.] .footer[ Imbens and Rubin (2015) *Causal Inference* ] --- class: title title-1 ## Stable Unit Treatment Value Assumption .box-inv-1[ **The potential outcomes for any unit do not vary with the exposures assigned to other units**, and, for each unit, there are no different forms or versions of each exposure level, which lead to different potential outcomes.] .footer[ Imbens and Rubin (2015) *Causal Inference* ] -- .box-1.medium[No Interference] --- class: title title-1 # No interference .box-inv-1.medium[This is a strong assumption!] -- .box-1[What if *you* eating ice cream made me happy?] -- .box-1[What if *me* eating ice cream in front of you when you didn't have any made you angry?] -- .box-inv-1.medium[These are examples of **interference**!] --- class: title title-1 # Vaccines: a classic interference example .box-inv-1.medium[If everyone else is immunized with a perfect vaccine, your immunization will have no effect] -- .box-1.medium[In these cases, we can] -- .box-inv-1[define the **unit** more broadly, for example a community, hospital, school, etc] -- .box-inv-1[limit the number of units assigned to a particular exposure] --- class: title title-1 ## Stable Unit Treatment Value Assumption .box-inv-1[ The potential outcomes for any unit do not vary with the exposures assigned to other units, and, for each unit, there are no different forms or versions of each exposure level, which lead to different potential outcomes.] .footer[ Imbens and Rubin (2015) *Causal Inference* ] --- class: title title-1 ## Stable Unit Treatment Value Assumption .box-inv-1[ The potential outcomes for any unit do not vary with the exposures assigned to other units, and, for **each unit, there are no different forms or versions of each exposure level, which lead to different potential outcomes.**] .footer[ Imbens and Rubin (2015) *Causal Inference* ] --- class: title title-1 # Exposure assignment .box-1.medium[ `\(Y_{me}^{obs}\)`(π¦) = π ] -- <br> .box-inv-1[I decided to eat ice cream because I was feeling quite grumpy and thought it might make me feel better! It did!] --- class: title title-1 # Exposure assignment .box-1.medium[ `\(Y_{you}^{obs}\)`(no π¦) = π ] -- <br> .box-inv-1[You decided not to eat ice cream because you were feeling fine. After you were happy!] --- class: title title-1, center # <svg style="height:0.8em;top:.04em;position:relative;" viewBox="0 0 640 512"><path d="M624 416H381.54c-.74 19.81-14.71 32-32.74 32H288c-18.69 0-33.02-17.47-32.77-32H16c-8.8 0-16 7.2-16 16v16c0 35.2 28.8 64 64 64h512c35.2 0 64-28.8 64-64v-16c0-8.8-7.2-16-16-16zM576 48c0-26.4-21.6-48-48-48H112C85.6 0 64 21.6 64 48v336h512V48zm-64 272H128V64h384v256z"/></svg> Application Exercise <br><br> .huge[ [github.com/sta-679-s22](https://github.com/sta-679-s22) ] --- class: title title-1 # Exposure assignment .box-inv-1[Understanding the **assignment mechanism** is crucial for understanding the causal effect] -- .box-1[Assignment probability must be **individualistic** (your covariates / potential outcomes can't influence my assignment)] -- .box-1[All units must have **non-zero** probability for all exposures] -- .box-1[Assignment **cannot** depend on the potential outcomes] --- class: title title-1 # Exposure assignment .box-inv-1[Understanding the **assignment mechanism** is crucial for understanding the causal effect] .box-1[Assignment probability must be **individualistic** (your covariates / potential outcomes can't influence my assignment)] .box-2[All units must have **non-zero** probability for all exposures] .box-2[Assignment **cannot** depend on the potential outcomes] --- class: title title-1 # Exposure assignment .box-inv-1[Understanding the **assignment mechanism** is crucial for understanding the causal effect] .box-2[Assignment probability must be **individualistic** (your covariates / potential outcomes can't influence my assignment)] .box-1[All units must have **non-zero** probability for all exposures] .box-2[Assignment **cannot** depend on the potential outcomes] --- class: title title-1 # Exposure assignment .box-inv-1[Understanding the **assignment mechanism** is crucial for understanding the causal effect] .box-2[Assignment probability must be **individualistic** (your covariates / potential outcomes can't influence my assignment)] .box-2[All units must have **non-zero** probability for all exposures] .box-1[Assignment **cannot** depend on the potential outcomes] --- class: title title-1 # Notation .box-inv-1.medium[ .huge[ `\(\mathbf{Y}(0), \mathbf{Y}(1)\)` ] ] -- <br> .box-1[ vectors of potential outcomes where the `\(i\)`th is equal to `\(Y_i(0)\)` / `\(Y_i(1)\)` ] --- class: title title-1 # Notation .box-inv-1.medium[ .huge[ `\(\mathbf{X}\)` ] ] -- .box-1[ vector of exposure assignment ] --- class: section-title-1 title-1 middle # Causal inference is fundamentally a missing data problem .footer[Rubin (1974) Journal of Educational Psychology doi:10.1037/h0037350] --- class: title title-1 # Outcomes for an individual .box-inv-1[ `\(Y_i^{obs}= Y_i(X_i)=\begin{cases}Y_i(0)&\textrm{if }X_i=0\\Y_i(1)&\textrm{if }X_i = 1\end{cases}\)` ] -- <br> .box-1[ `\(Y_i^{mis}= Y_i(1-X_i)=\begin{cases}Y_i(1)&\textrm{if }X_i=0\\Y_i(0)&\textrm{if }X_i = 1\end{cases}\)` ] --- class: title title-1 # Outcomes for an individual .box-inv-1[ `\(Y_i(0)= \begin{cases}Y_i^{mis}&\textrm{if }X_i=1\\Y_i^{obs}&\textrm{if }X_i = 0\end{cases}\)` ] <br> -- .box-1[ `\(Y_i(1)= \begin{cases}Y_i^{mis}&\textrm{if }X_i=0\\Y_i^{obs}&\textrm{if }X_i = 1\end{cases}\)` ] --- class: section-title-1 title-1 middle # Causal inference is fundamentally a missing data problem .footer[Rubin (1974) Journal of Educational Psychology doi:10.1037/h0037350] -- .box-inv-1[If we impute the missing outcomes, we can know the value of any causal estimand] --- class: title title-1 # Assignment mechanism .box-inv-1.medium[ `\(P(\mathbf{X}|\mathbf{Z}, \mathbf{Y}(0), \mathbf{Y}(1))\)` ] -- <br> .box-1[ What is `\(\mathbf{Z}\)` ] -- <br> .box-inv-1[ A matrix of **pre-exposure** covariates ] --- class: title title-1 # Assignment mechanism .box-inv-1.medium[ `\(P(\mathbf{X}|\mathbf{Z}, \mathbf{Y}(0), \mathbf{Y}(1))\)` ] -- .box-1[such that] -- .box-inv-1[ `$$\sum_{X\in\{0,1\}^N}P(\mathbf{X}|\mathbf{Z}, \mathbf{Y}(0), \mathbf{Y}(1)) =1$$` for all `\(\mathbf{Z}\)`, `\(\mathbf{Y}(0)\)`, and `\(\mathbf{Y}(1)\)` ] --- class: title title-1 # Assignment mechanism .box-inv-1[ In words: this is the probability that a particular value for the full assignment will occur -- like person 1 gets assigned to the exposure, 2 & 3 to control, 4 to exposure, and so on. The probabilities across the full set of `\(2^N\)` possible assignment vectors must sum to 1. ] -- .box-1.medium[This is **not** the probability that a particular person will be assigned a particular exposure] --- class: title title-1 # Unit Assignment Probability .box-inv-1[ `\(P_i(\mathbf{Z}, \mathbf{Y}(0), \mathbf{Y}(1))=\sum_{\mathbf{X}:X_i=1}P(\mathbf{X}|\mathbf{Z}, \mathbf{Y}(0),\mathbf{Y}(1))\)` ] -- <br> .box-1[ You can get this by summing across all possible assignment vectors, `\(\mathbf{X}\)` where `\(X_i=1\)` ] --- class: section-title-1 title-1 middle # Propensity score: The average unit assignment probability for units where `\(X_i=x\)` .footer[Imbens and Rubin (2015) Causal Inference] --- class: title title-1 # Propensity Score .box-inv-1.medium[ `\(e_i(x)=\frac{1}{N(x)}\sum_{i: X_i=x}P_i(\mathbf{Z}, \mathbf{Y}(0), \mathbf{Y}(1))\)` ] ---